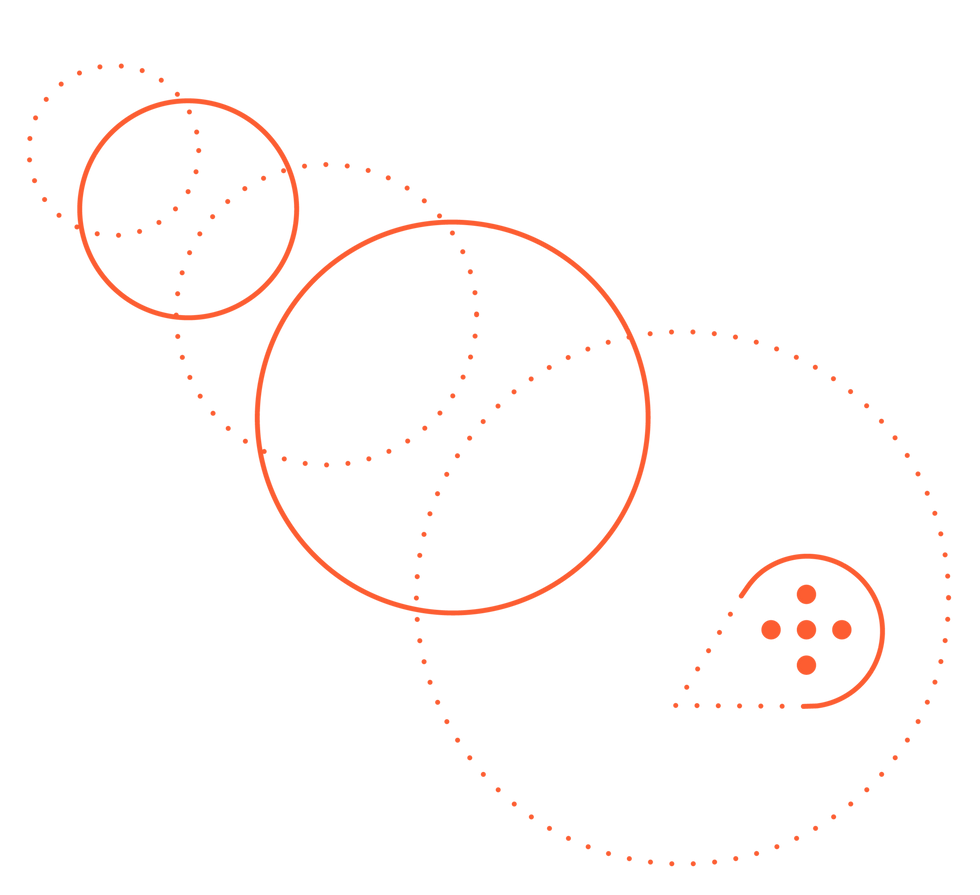
As AI transforms practically every industry, including the financial sector, concerns about potential biases and unintended consequences in AI models are rising. While AI holds immense potential, it's not perfect.
Imagine a loan application being rejected due to an algorithm misinterpreting someone’s financial data. Such errors, though unintended, can have profound impacts on individuals' lives and raise critical questions about the reliability and fairness of AI systems. It can also lead to lawsuits, financial losses, and regulatory and reputational damages.
Understanding and mitigating these unintentional AI failures is vital in an era where high-risk decisions are increasingly automated. By examining real-world examples and dissecting the underlying causes, we aim to shed light on what went wrong and how you can prevent it from happening to your organization in the future.
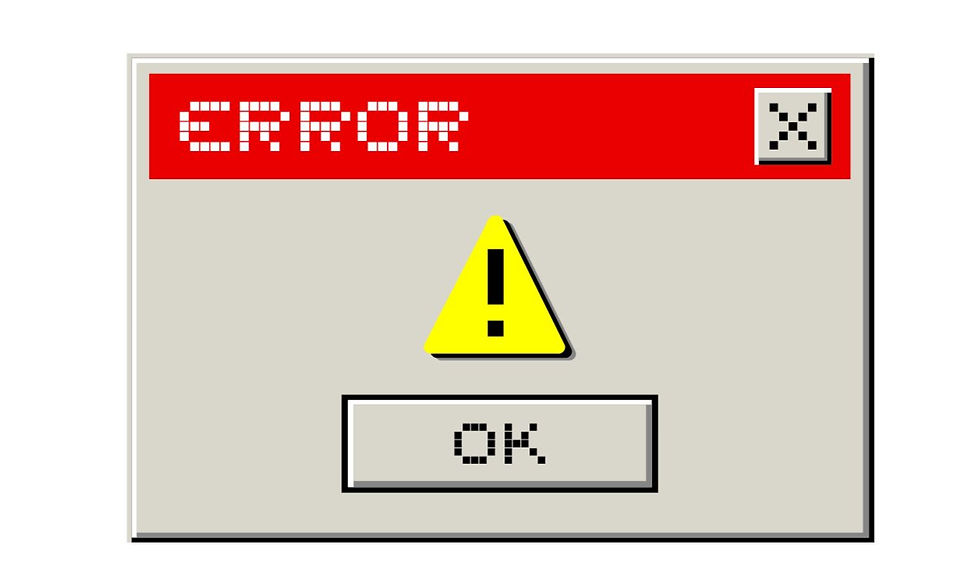
Lesson 1: Sensitive Features Can Still Be Correlated Even When They Are Not Seen By the Model
In November 2019, Apple and Goldman Sachs received complaints from Apple Card applicants about a potential gender bias in the credit assessment algorithm. The applicants claimed men were granted much higher credit limits than women with equal credit qualifications.
As a result, the New York Department for Financial Services launched an investigation into the discrimination claim. Meanwhile, Goldman Sachs, the bank issuing the card, responded that the algorithm couldn’t be biased because it doesn’t even use gender as an input.
When it comes to ensuring models are fair, it often seems that removing sensitive features like race and gender will prevent the model from being biased. However, even without explicitly inputting these attributes, customer data can still exhibit correlations, potentially leading to unintended bias.
By intentionally ignoring a critical factor like gender, Goldman Sachs made it more challenging to detect, prevent, and correct bias related to it.
This could have been prevented if they had deeper insights into the data’s behavior and understood which significant features would correlate to an applicant’s gender.
Lesson 2: Biases Need to Be Addressed and Monitoring Is Key
Navy Federal Credit Union is facing a class-action lawsuit over claims that its lending algorithm unfairly denied loans to people of color. A CNN report found that in 2022, 77% of white applicants were approved for loans, compared to only 48% of black applicants, even when they had strong financial profiles. This highlights the broader issue of biased lending models.
Reducing biases in algorithmic decision-making is essential for fostering fairness and equality. Biases embedded in algorithms can perpetuate systemic inequalities, disadvantaging marginalized groups. Adopting alternative methods, such as real-time data and comprehensive risk assessment methodologies, allows organizations to more accurately gauge risk and opportunity, ensuring fair treatment for all stakeholders. This approach promotes inclusivity and strengthens trust in regulatory compliance and ethical practices.
As algorithms evolve with new data in operational settings, continuous monitoring becomes paramount to mitigate risks of drifts and biases. Understanding when the models need to be updated or discontinued helps in maintaining their accuracy and relevance. Implementing robust monitoring frameworks ensures that any deviations or biases are promptly detected and corrected. This proactive approach not only enhances the reliability of decision-making processes but also fosters transparency and accountability within the regulatory and operational frameworks of industries facing heightened scrutiny.
Don’t Make the Same Mistakes
As AI reshapes various sectors, particularly in finance, the stakes around biases and unintended consequences in AI models are higher than ever. Examples like the Apple Card controversy and Navy Federal's lending algorithm disparities serve as stark reminders of the potential legal, financial, and reputational risks involved.
Organizations must take decisive action to protect themselves from these risks. This means rigorously scrutinizing data for hidden correlations that could unknowingly influence decisions, adopting alternative metrics like real-time data for more equitable assessments, and establishing robust monitoring systems to swiftly detect and correct biases.
It might seem like a lot of extra work, but that's exactly where we excel. At Citrusx, we make these processes simpler to save you time and guarantee the accuracy, robustness, and transparency of your models. Our solution includes online monitoring to promptly alert you when the model should be updated or discontinued, due to drifts in performance. Citrusx also automatically runs a full model validation process. Moreover, we ensure compliance with our comprehensive reporting system, delivering customized reports to all stakeholders in your pipeline. This keeps everyone informed, enabling better business decisions and minimizing risks to your organization.
Learn more about how Citrusx can help you by booking a demo with our team.
Share
Share
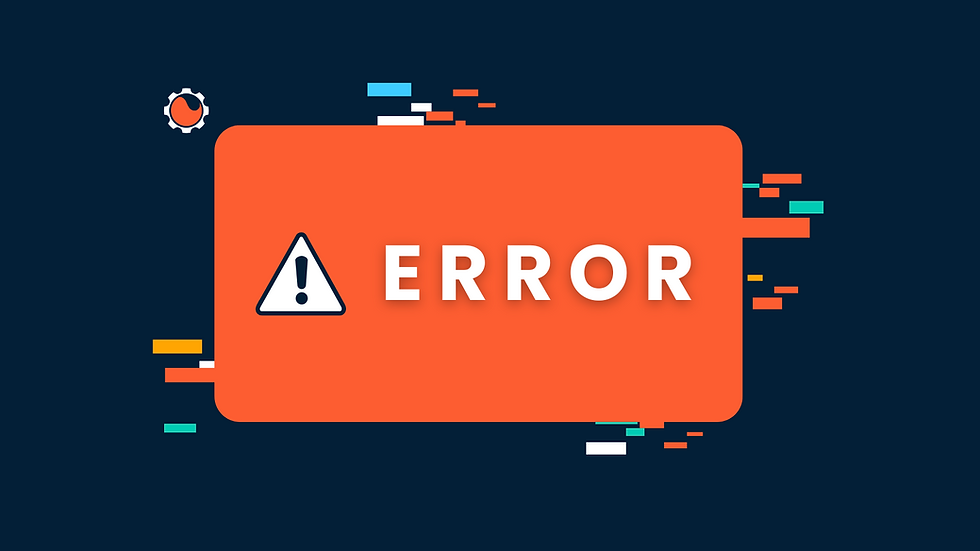
Lessons in Unintentional AI Failures: Protect Your Business from AI Mistakes
Citrusx